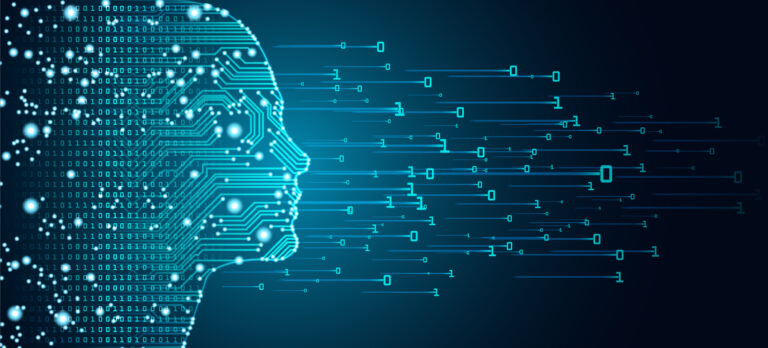
Can liver fibrosis be staged by deep learning techniques?
This pilot study aims to investigate whether liver fibrosis can be staged by deep learning techniques based on CT images. It included CT examinations of patients who underwent dynamic contrast-enhanced CT for evaluations of the liver and for whom histopathological information regarding liver fibrosis stage was available. Some additional images for training data were generated by rotating or parallel shifting